Reducing Human Bias, Optimizing Supply Chain Forecasting—An Exclusive Interview with MIT Supply Chain Expert Jeff Baker
-
2024-07-08
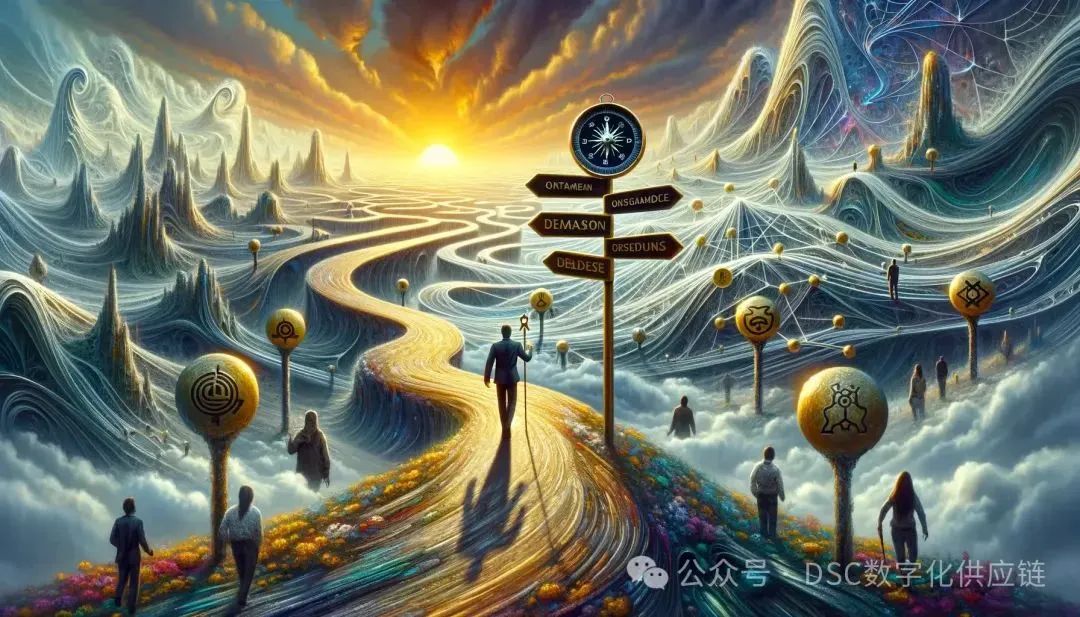
Prevalence of Forecasting Bias
Three Key Rules for Optimizing Forecasts
Faced with the pervasive issue of forecast bias, Jeff Baker, leveraging his years of research and practical experience, has distilled three key rules for optimizing forecasts. These rules not only provide clear directions for identifying and quantifying biases but also offer valuable guidance on balancing statistical models with human judgment.
Rule 1: Exercise Caution in Adjusting Well-Performing Forecasts
Jeff Baker humorously remarks, “In the realm of forecasting, we often say ‘Don’t just stand there, do something,’ but sometimes the opposite is true—‘Don’t do something, just stand there’ is the wiser choice for forecasts that are already performing well.”
He further explains that when statistical forecasting models are producing high-confidence, low-bias results, additional manual adjustments may introduce the risk of “overfitting.” This is especially true when these adjustments lack robust statistical backing. Subjective assumptions about seasonal patterns, overreactions to low-probability events, and interference from various vested interests can all lead to deviations from the model’s optimal output.
Therefore, Jeff Baker recommends asking three critical questions before making any manual adjustments: First, how high is the confidence level of the existing model? Is its error rate within an acceptable range? Second, have the insights I possess already been fully considered by the model? Is there solid evidence supporting my judgment? Finally, do the benefits of the adjustment outweigh the potential costs? Will it introduce new risks? If the answer to all three questions is negative, it is best to refrain from altering the forecast results.
In practice, Jeff Baker has observed that manual adjustments to demand forecasts are particularly susceptible to overconfidence and herd mentality. “Sales personnel tend to give optimistic forecast results because they do not want to miss any sales opportunities or be blamed for underestimating demand. When optimistic expectations become a consensus within the team, it requires significant courage to go against the tide and lower the forecast value. This is one reason why upward adjustments to forecasts are more frequent than downward adjustments.”
By adhering to these principles, companies can mitigate the negative impact of manual adjustments and maintain the integrity and reliability of their forecasts.
Rule 2: Quantify Bias and Variance
Even well-considered manual adjustments can introduce subtle biases that are difficult to detect. To measure these biases more accurately, Jeff Baker recommends using the statistical indicators of Bias and Variance to quantify forecast errors and further analyze their nature and sources.
From a statistical perspective, error can be decomposed into two components: Bias and Variance. Bias reflects the systematic deviation between the forecasted values and the actual values, while Variance reflects the volatility of the forecasted values themselves. In supply chain forecasting, Jeff Baker found that manual adjustments are more likely to introduce systematic Bias, which often goes unnoticed.
For example, sales personnel tend to adjust system forecast values upward to align them more closely with annual sales targets, rarely considering whether this upward adjustment has sufficient objective justification. Jeff Baker humorously refers to this behavior as “credit grabbing,” because if the sales results exceed expectations, the sales department often claims the credit, but if the results fall short, the blame is placed on the inaccuracy of the system’s forecast. Over time, this leads to sales forecast values systematically exceeding actual demand, with few recognizing this bias.
How can one determine if a forecast adjustment is overly optimistic, overly pessimistic, or within a reasonable range of uncertainty? Jeff Baker proposes a simple yet practical method: “We can define the difference between the adjustment value and the statistical forecast value as the adjustment amount and compare them with the actual values afterward. If the adjustment amount often exceeds the actual forecast error and the direction is consistent, it indicates over-adjustment; conversely, if the adjustment amount is often less than the actual forecast error, it indicates under-adjustment; if the adjustment amount is comparable to the forecast error and balances out in both directions, it is likely within the reasonable range of uncertainty.”
By regularly reviewing and analyzing changes in Bias and Variance, the forecasting team can promptly identify and correct systematic biases in manual adjustments, thereby continually improving the accuracy of their judgments and ultimately achieving more precise forecasting.
Rule 3: Use the "Best Case, Worst Case, Most Likely Case" Triangular Distribution to Express Uncertainty
When attempting to make accurate predictions about the future, it's often challenging to provide a definitive number, but relatively easier to describe a rough range or interval. Based on this observation, Jeff Baker proposed using the "Best Case, Worst Case, Most Likely Case" triangular distribution to express the uncertainty in human judgment.
He explains, “When I communicate with the sales and marketing departments and ask them to estimate the demand for a new product, they often provide a relatively optimistic target figure. However, this figure hides many uncertainties, such as changes in competitors’ market strategies and shifts in consumer preferences. To better reveal these uncertainties, I further ask them for their predictions of the worst-case and most likely scenarios and use a triangular distribution to model and analyze these scenarios.”
It is important to note that setting up these three scenarios requires close cooperation and communication across departments. Jeff Baker emphasizes that this is not a task that can be completed independently by any single department. It requires the collaboration and brainstorming of multiple departments, including supply chain, sales, marketing, and finance. “I invite the relevant departments to give their judgments on the three key points of the triangular distribution from their professional perspectives. During this process, we examine each party’s assumptions, discuss market opportunities and potential risks, and ultimately form a comprehensive and balanced triangular distribution model.”
Once the triangular distribution model is established, Jeff Baker further introduces the Monte Carlo simulation method to generate multiple possible demand scenarios and their corresponding probability distributions. “Through random sampling, we can simulate hundreds of potential demand outcomes, each based on a probability-weighted random combination. This way, the originally single forecast value is transformed into a forecast distribution with multiple possibilities. Our judgment of the future market expands from a discrete point to a continuous interval range. Based on such a probability distribution, we can more accurately calculate expected demand, confidence intervals, and other important indicators, thus providing richer data support for risk assessment and inventory optimization.”
Jeff Baker’s three rules not only deeply reveal the essence of the forecasting bias problem but also provide valuable operational guidelines for supply chain management practitioners. However, to truly implement these rules, we must face and overcome human limitations, break down departmental silos, establish effective incentive mechanisms and organizational cultures, and cultivate a positive forecast correction mechanism. This will be a topic we need to explore further in subsequent chapters.
Overcoming Bias to Build Accurate Supply Chain Forecasting
Designing Reasonable Incentive Mechanisms
To truly eliminate biases in forecasting, relying solely on technical means is insufficient. It also requires addressing human motivations, coordinating interests from all parties, and establishing a positive incentive framework. Jeff Baker once assisted a consumer goods company in restructuring their sales forecasting process. Through data analysis, he discovered that sales forecasts often astonishingly aligned with sales targets. Upon deeper investigation, he found that sales managers were concerned that forecasted numbers could affect their sales targets and performance evaluations, leading them to provide conservative "safe" forecasts. To address this issue, Jeff Baker and the company executives jointly designed a new performance assessment method: incorporating forecast accuracy into the sales team's performance metrics, balanced with sales task completion rates. Simultaneously, they established a tolerance mechanism for sales forecasts, allowing for a certain range of deviation to alleviate concerns about being completely candid. This initiative achieved significant results, as sales managers began to pay closer attention to forecast accuracy.
Promoting Cross-Departmental Understanding and Trust
To fundamentally resolve the issue of forecasting biases, it is essential to break down departmental silos and establish a shared vision. Jeff Baker suggests promoting mutual understanding of each department's professional perspectives through methods such as cross-departmental rotations and joint workshops. He emphasizes the need for a dialectical unity of logic and intuition in forecasting. For example, sales personnel can be invited to the supply chain department to learn about the logic of demand analysis, while data analysts can spend time in the marketing department to understand how to capture customer psychology. Additionally, organizing forecasting competitions involving multiple departments can be an effective way to build rapport and trust through friendly competition.
Building a Resilient Supply Chain: Embracing Uncertainty and Enhancing Forecasting Capabilities
As market uncertainty increases, relying solely on forecasting has become insufficient to cope with rapidly changing challenges. More and more companies are focusing on cultivating supply chain resilience, aiming not only to improve forecast accuracy but also to build a flexible, adaptable supply chain. In this process, digital technology is important, but even more crucial is the reshaping of decision-making processes and the creation of a learning organization.
Supply Chain Transparency: Breaking Down Information Silos
Information asymmetry within the supply chain is a breeding ground for forecasting errors. Jeff Baker discovered that many forecasting mistakes stem from poor information sharing between departments and a lack of an overall perspective. To break down information barriers, companies need to build a shared digital platform that connects data sources from all supply chain links, including point-of-sale data, logistics tracking data, and supplier inventory data. This platform should provide data visualization and collaboration tools to facilitate real-time communication and alignment of expectations among departments. By achieving supply chain transparency, companies can gain real-time insights into the supply chain's operational status and adjust their plans accordingly.
Developing Multi-Scenario Contingency Plans: Turning Uncertainty into Opportunity
The core of supply chain resilience lies in the ability to respond quickly to unexpected changes. To achieve this, companies need to develop multi-scenario contingency plans in advance, addressing key uncertainties that affect demand. These plans should consider not only negative impacts but also potential opportunities. For example, if market demand exceeds expectations, companies can quickly scale up production; if there is a supply shortage, they can find alternative sources; if oil prices fluctuate significantly, they can optimize transportation modes. By developing multi-scenario contingency plans, companies can respond calmly and turn uncertainty into opportunity.
Supply Chain Talent Development: From Demand Response to Demand Creation
Future supply chain managers need to not only accurately predict demand but also proactively create demand and lead trends. This sets higher requirements for practitioners' skills. Jeff Baker believes that supply chain managers must possess a global perspective, digital thinking, and innovation awareness as core skills. To cultivate these skills, companies need to establish a dual-path talent development system: setting up formal job rotation mechanisms to allow outstanding talent to gain experience in different supply chain positions, and assigning mentors to innovation projects to provide talent with opportunities to challenge themselves and showcase their abilities. Additionally, companies should foster a culture of continuous learning, encouraging employees to maintain an open, humble, and curious mindset, constantly absorbing new knowledge and inspiration.
Embracing the Unknown: A Closing Thought
Jeff Baker concluded the interview with his favorite quote: "All models are wrong, but some are useful." This seemingly paradoxical statement captures the essence of forecast optimization. Indeed, no forecasting model can be perfect because the future always holds "unknown unknowns." However, if we accept this fact and continuously learn and iterate while using models, we can find a path that approaches the truth and benefits decision-making. This is the wisdom and responsibility that supply chain professionals should embody.
===========
This content is reprinted from: WeChat Official Account - DSC Digital Supply Chain. The article represents the author's views only. If you have any suggestions or questions, please contact me.
DSC (Digital Supply Chain) aims to bring together top domestic digitalization and supply chain experts to discuss professional issues and cutting-edge topics in the field of supply chain, exploring the development direction of supply chains in the digital realm.
END

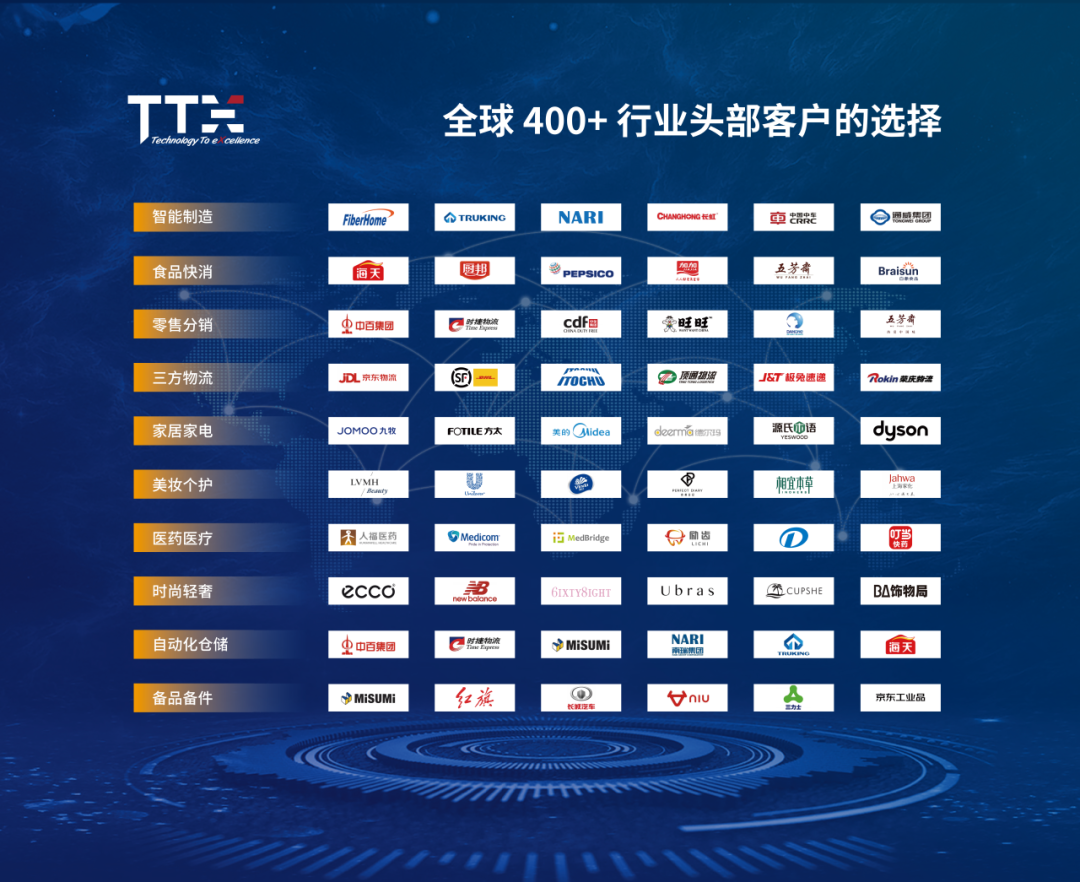
Share